I’ve just returned from the remarkable Workshop for Instruction in Library Use 2023 (WILU). These types of workshops never fail to ignite my enthusiasm and inspire my work in libraries. This year’s WILU was no exception. One session, in particular, stood out as the crowd favourite: “Imagining Instructional Practices in the Context of Generative AI.” Engaging in an open dialogue about the capabilities and impact of generative AI tools such as ChatGPT, Bing, and Google AI was truly invigorating, especially considering the implications for our profession.
The advent of generative AI has created intense conversations within the Higher Education realm, evoking a wide range of reactions, from cautious contemplation to outright alarm. While many succumb to a pessimistic outlook, I maintain a more optimistic perspective, seeing generative AI tools as an opportunity for us to enhance and underscore our value within academic institutions. Of course, it’s crucial to acknowledge that this viewpoint is contingent upon the current state of generative AI.
The current state of generative AI
Anticipating the unavoidable influx of questions about these types of tools, I undertook a short investigation into the limitations of the existing free version of ChatGPT, commonly utilized by the majority of students. Anecdotal accounts regarding students’ utilization of ChatGPT have been widespread, and I have personally witnessed students using it. Within the realm of library expertise, a critical concern that arises with the present state of generative AI is its tendency to generate inaccurate citations for articles. This is known as AI hallucinations.
I’ve tested ChatGPT in the past and I will admit, it’s not great at creating real citations. But how wrong can it be? I wanted to find out. I decided to do what any researcher would do, I ran a test.
The full results and Google Sheets pages can be found here.
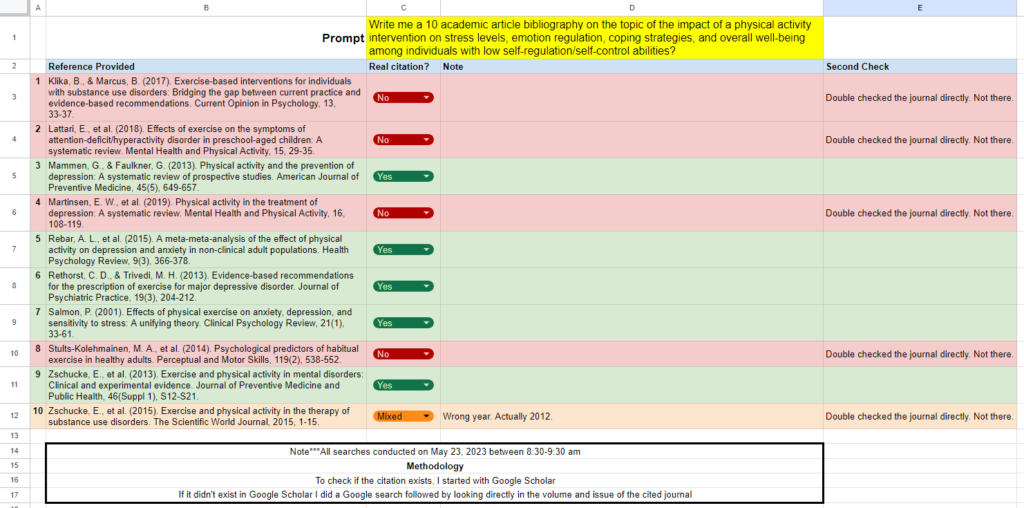
Methodology
In this review of ChatGPT’s capabilities for producing an initial bibliography on specific topics, a straightforward methodology was employed to analyze the results. The following steps were undertaken:
- Execution of 10 prompts consecutively within the same “conversation” to ensure consistency in using the same model. These prompts can be found in the designated section of the document.
- The responses generated by ChatGPT were examined, and 10 citations were extracted and transferred to Google Sheets. Each prompt’s citations were organized in separate worksheets (link provided).
- Each citation was carefully scrutinized to determine its authenticity, classifying it as either “Yes” (real), “No” (critical errors), or “Mixed” (concerns but seemingly real).
- For citations identified as “fake” or “mixed,” a secondary search was conducted to verify their validity. This involved directly accessing the referenced journal and cross-referencing the provided date, volume, issue, and pagination with the citation.
- The analysis solely focused on identifying whether the articles generated by ChatGPT were real, fake, or mixed. It did not assess the content or relevance of the articles to the given prompts.
By employing this methodology, a comprehensive evaluation of ChatGPT’s citation accuracy was conducted, specifically focusing on the authenticity of the generated articles.
Findings
To conduct my test, I executed a series of 10 ChatGPT prompts, each requesting a bibliography of 10 scholarly or academic articles pertaining to a specific topic (e.g., “Provide me with a bibliography of 10 academic articles on the subject of…”). The topics encompassed a wide range of subjects, including psychology, climate change, immigration, armed conflict, culture, and economics, among others.
In general, ChatGPT exhibited limitations when it came to generating authentic citations. Out of the 100 article citations generated, only 34 were found to be genuine citations. A significant portion, 55 citations, were entirely fabricated, while 11 fell into the category of “mixed” citations. Mixed citations possessed certain elements that were genuine, such as the article title and authors, but may have falsified details such as the journal name, year of publication, volume/issue number, or pagination.

The Good
1 of the prompts generated nearly complete correct citations (8/10 correct, 2/10 fake).
- Prompt 9: Write me a 10 academic article bibliography on the topic of self expression and fashion and how those impact someone’s identity construction
The Bad
3 of the prompts generated completely fake or mixed citations, those were:
- Prompt 3: Write me a 10 academic article bibliography on the topic of Japan’s use of soft power to influence its impact on western countries (9/10 fake, 1/10 mixed)
- Prompt 6: Write me a 10 academic article bibliography on the topic of Afghani refugees who are establishing themselves in Canada post U.S. withdrawal (10/10 fake)
- Prompt 7: Write me a 10 academic article bibliography on the topic of public transportation in Canada and how recent conversations surrounding the importance of transit infrastructure are reigniting the public’s interest in high-quality and affordable public transportation (9/10 fake, 1/10 mixed)
The Ugly
The remaining 6 prompts generated a mix of real and fake citations and this is where things get ugly (Prompts 1, 2, 4, 5, 8, 10).
If we lived in a world of black and white, knowing whether something was generating ALL fake or ALL real citations would make evaluating a tool very easy. However, the current state of ChatGPT’s ability to create accurate and real citations is very concerning but also offers us a unique opportunity to sell the need for greater information literacy skills to students and faculty. In a future post, I plan on turning this exercise into an activity for students to complete in the classroom.
Where does ChatGPT excel?
In evaluating the ability of ChatGPT to generate real and accurate citations, I was expecting the tool to generate fake journals. I was pleasantly surprised to find that ChatGPT was finding real journals and that those journals (on first inspection) appeared to be valuable and apparently relevant to the topic at hand.
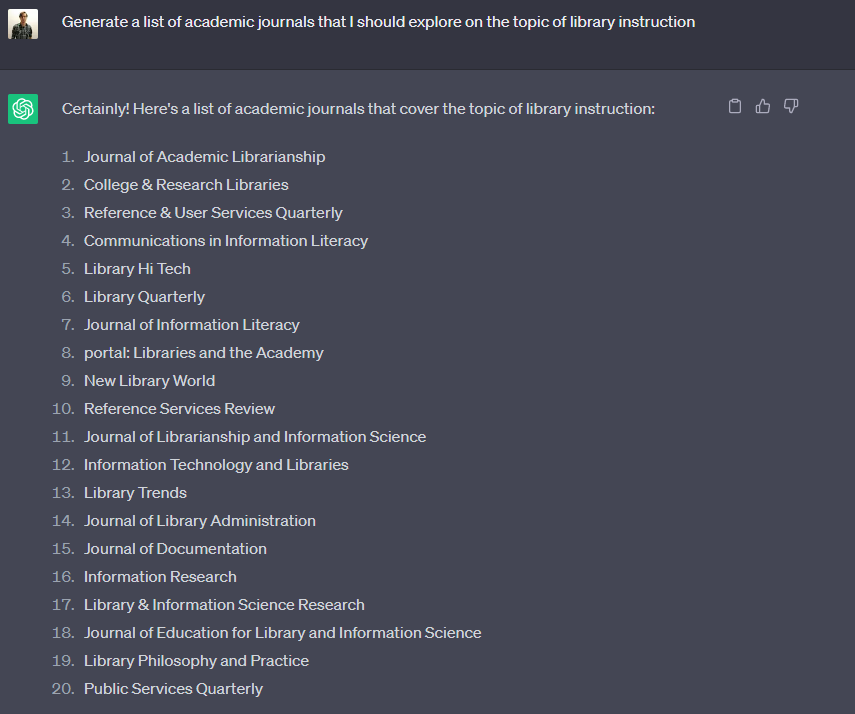
For example, as someone interested in library instruction, I prompted ChatGPT for “a list of academic journals that I should explore on the topic of library instruction”. To which it responded with a fantastic list of journals I could browse. I could then consider browsing those titles for relevant articles, or create alerts when journal articles are written using specific keywords. Similarly, I could see this being valuable to researches looking to conduct a knowledge synthesis looking to hand browse some of the more prominent journals on a subject area.
Future Research
In the future, I plan to employ a comparable methodology to adopt a more informal approach with ChatGPT, departing from a strictly “scholarly” perspective. Further investigation could be conducted in future research to analyze the actual citations in relation to their relevance to the given prompts.
Update (June 6, 2023)
Since conducting my own test with ChatGPT I discovered Dr. David Wilkinson’s YouTube video where he goes through the same methodology that I did. I was unaware of Dr. Wilkinson’s research before conducting my own test but we followed a similar methodology. Check out his work on generative AI and large language models.
Header photo by Emily Morter on Unsplash